Case Study: Medical Dataset Licensing
Transforming Pediatric & OB-GYN Care Through Precision Data Curation & Annotation Training
Unlocking the Power of Medical Data: Comprehensive Data Curation, De-identification, ICD-10 CM, and Annotation for Superior AI Model Training.
Project Overview
Shaip partnered with a leading healthcare AI company to curate and annotate high-quality, de-identified medical datasets for training advanced NLP models. The project focused on Pediatrics and OB-GYN specialties, delivering outpatient records annotated with ICD-10 CM codes via a robust API framework.
The dataset was structured to facilitate AI training on real-world healthcare documentation, enhancing model capability in understanding clinical narratives.
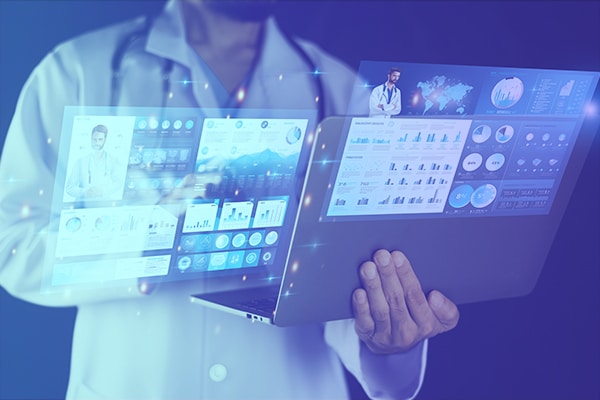
Key Stats
750 pages / ~300 outpatient records
Project Scope
Dataset Type | Specialty | Volume | Metadata Captured | Notes |
---|---|---|---|---|
Medical Notes | Pediatrics | 375 pages (~150 records) | File Name, Specialty, Document Type, Patient Class (Outpatient) | Includes Assessment / Plan sections |
OB-GYN | 375 pages (~150 records) | |||
Annotations | ICD-10 CM (2023) | Full Dataset | Code Mapping via API | Code validation by coders is out of scope |
Challenges
The project presented several critical challenges that required meticulous planning and execution:
Sourcing high-quality outpatient records exclusively from Pediatrics and OB-GYN specialties was challenging. Each document needed to include key clinical sections like Assessment and Plan to support accurate annotations.
Ensuring complete removal of all personally identifiable information (PII) while maintaining the medical context was essential for HIPAA compliance. This required detailed reviews to prevent any privacy breaches.
Applying precise ICD-10 CM (2023) codes via API was complex due to varied narrative styles and medical terminology. Consistency and accuracy in coding were critical to ensure reliable AI model training.
Capturing and validating metadata such as specialty, document type, and patient class without discrepancies was vital. Any mismatch could impact model training and data usability.
Ensuring all records were strictly outpatient added complexity, as many clinical documents may contain mixed patient classes or incomplete sections.
Meeting the 90% accuracy threshold demanded multi-level reviews to eliminate duplicates, validate specialty alignment, and ensure de-identification — with provisions for rework when needed.
Solution
Comprehensive Data Licensing & Annotation
- Licensed pediatric and OB-GYN outpatient records
- Ensured inclusion of critical sections: Chief Complaint, History, ROS, Assessment, Plan
- API-based ICD-10 CM annotation (2023 version)
De-identification & Compliance
- Replaced PHI with placeholders (PERSON_NAME, DATE, LOCATION, etc.)
- Ensured compliance with healthcare data privacy standards
Metadata Tagging
- Captured detailed metadata per file:
-
- File Name
- Specialty (Pediatrics or OB-GYN)
- Document Type (Follow-up, H&P, Consultation)
- Patient Class (Outpatient Only)
Quality Control
- Rigorous quality assessments with:
- No duplicate records
- Specialty match validation
- Outpatient-only check
- Metadata consistency check
- Replacement or correction of records below 90% accuracy threshold
Outcome
Shaip delivered a structured, annotated medical notes dataset that enabled the client to:
- Train AI models for accurate ICD-10 CM code prediction
- Enhance NLP capabilities in real-world healthcare scenarios
- Maintain compliance with privacy and regulatory standards
- Scale healthcare AI models across pediatrics and OB-GYN domains
Shaip’s structured approach to dataset curation and annotation exceeded our expectations. The accuracy, de-identification, and metadata precision have significantly strengthened our AI model training pipeline.