LiDAR Annotation Project for SmartCity Autonomous Vehicles
Project Overview
SmartCity, a rapidly growing metropolitan area, initiated an ambitious project to introduce autonomous vehicles (AVs) for public transportation. To ensure safe and efficient operation of these AVs, they needed a vast amount of accurately annotated LiDAR and camera data representing the city’s diverse environments. SmartCity partnered with Shaip, a leading data annotation company, to handle this crucial task.
Shaip was tasked with annotating 15,000 frames of sensor data collected from SmartCity’s streets. Each frame contained data from 3 Velodyne VLP-32C LiDARs and 4 high-resolution cameras, capturing a wide range of urban scenarios
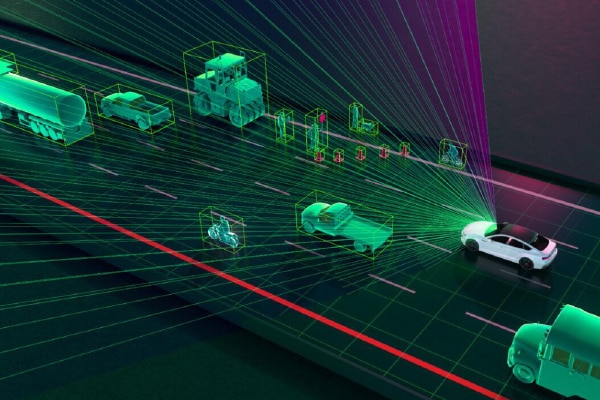
Challenges
The sheer amount of data and the requirement for both 2D and 3D annotations presented a significant challenge.
SmartCity's varied landscape, from dense urban centers to suburban areas, required adaptable annotation strategies.
Maintaining consistent object IDs across different sensors and multiple frames was crucial for training reliable AI models.
Ensuring all personal identifiable information was properly masked while preserving useful data.
SmartCity needed the project completed within 4 months to meet their AV deployment schedule.
Shaip’s Approach
Assembled a team of 50 experienced annotators, 10 quality controllers, and 3 project managers.
Developed proprietary software that integrated 2D and 3D annotation workflows, enhancing efficiency and consistency.
Conducted intensive training sessions on SmartCity's specific annotation requirements and privacy guidelines.
Utilized AI-assisted pre-annotation to speed up the process, especially for common objects like cars and pedestrians.
Outcome
- Completed the project in 3.5 months, two weeks ahead of schedule.
- Achieved 99.7% annotation accuracy, exceeding SmartCity’s expectations.
- Successfully annotated over 450,000 unique objects across all frames.
- Maintained consistent IDs for 98% of objects across multiple frames.
- Properly masked all license plates and faces, ensuring privacy compliance.
Conclusion
Shaip’s successful execution of this large-scale LiDAR annotation project played a pivotal role in SmartCity’s autonomous vehicle initiative. The project demonstrated the importance of combining skilled human annotators with advanced AI-assisted tools to handle complex, multi-sensor data annotation tasks efficiently and accurately.
The high-quality annotated data allowed SmartCity to train their AV systems more effectively, reducing the time needed for real-world testing by 30%. The consistent and accurate annotations particularly improved the AVs’ object tracking and prediction capabilities in complex urban environments.