Case Study: Conversational AI
Over 3k hours of Data Collected, Segmented & Transcribed to build ASR in 8 Indian languages
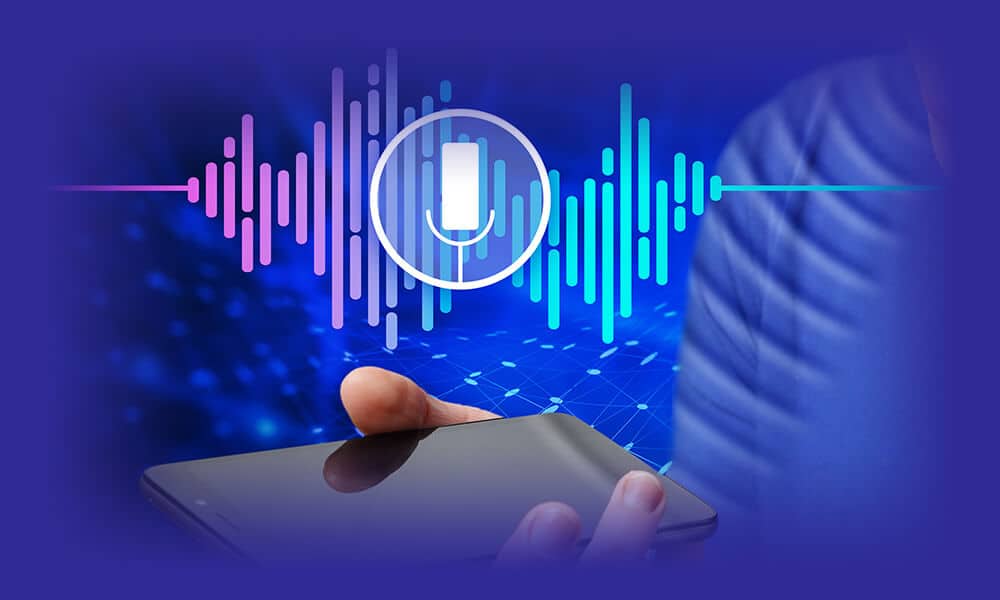
BHASHINI, India’s AI-driven language translation platform, is a vital part of the Digital India initiative.
Designed to provide Artificial Intelligence (AI) and Natural Language Processing (NLP) tools to MSMEs, startups, and independent innovators, the Bhashini platform serves as a public resource. Its goal is to promote digital inclusion by enabling Indian citizens to interact with the country’s digital initiatives in their native languages.
Additionally, it aims to significantly expand the availability of internet content in Indian languages. This is especially targeted towards areas of public interest such as governance and policy, science and technology, etc. Consequently, this will incentivize citizens to use the internet in their own language, promoting their active participation.
Harness NLP to enable a diverse ecosystem of contributors, partnering entities and citizens for the purpose of transcending language barriers, thereby ensuring digital inclusion & empowerment
Real World Solution
Unleashing the Power of Localization with Data
India needed a platform that would concentrate on creating multilingual datasets and AI-based language technology solutions in order to provide digital services in Indian languages. To launch this initiative, Indian Institute of Technology, Madras (IIT Madras) partnered with Shaip to collect, segment and transcribe Indian language datasets to build multi-lingual speech models.
Challenges
To assist the client with their Speech Technology speech roadmap for Indian languages, the team needed to acquire, segment and transcribe large volumes of training data to build AI model. The critical requirements of the client were:
Data Collection
- Acquire 3000 hours of training data in 8 Indian languages with 4 dialects per language.
- For each language, the supplier will collect Extempore Speech and
Conversational Speech from Age Groups of 18-60 years - Ensure a diverse mix of speakers by age, gender, education & dialects
- Ensure a diverse mix of recording environments as per Specifications.
- Each audio recording shall be at least 16kHz but preferably 44kHz
Data Segmentation
- Create speech segments of 15 seconds & timestamp the audio to the milliseconds for each given speaker, type of sound (speech, babble, music, noise), turns, utterances, & phrases in a conversation
- Create each segment for its targeted sound signal with a 200-400 millisecond padding at start & end.
- For all segments, the following objects must be filled i.e., Start Time, End Time, Segment ID, Loudness Level, Sound Type, Language code, Speaker ID, etc.
Data Transcription
- Follow details transcription guidelines around Characters and Special Symbols, Spelling and Grammar, Capitalization, Abbreviations, Contractions, Individual Spoken Letters, Numbers, Punctuations, Acronyms, Disfluent, Speech, Unintelligible Speech, Non-Target Languages, Non-Speech etc.
Quality Check & Feedback
- All recordings to undergo quality assessment & validation, only validated speech to be delivered
Solution
With our deep understanding of conversational AI, we helped the client collect, segment and transcribe the data with a team of expert collectors, linguists and annotators to build large corpus of audio dataset in 8 Indian languages
The scope of work for Shaip included but was not limited to acquiring large volumes of audio training data, segmenting the audio recordings in multiple, transcribing the data and delivering corresponding JSON files containing the metadata [SpeakerID, Age, Gender, Language, Dialect,
Mother Tongue, Qualification, Occupation, Domain, File format, Frequency, Channel, Type of Audio, No. of speakers, No. Of Foreign Languages, Setup used, Narrowband or Wideband audio, etc.].
Shaip collected 3000 hours of audio data at scale while maintaining desired levels of quality required to train speech technology for complex projects. Explicit Consent Form was taken from each of the participants.
1. Data Collection
2. Data Segmentation
- The audio data that was collected was further bifurcated into speech segments of 15 seconds each and timestamped to the milliseconds for each given speaker, type of sound, turns, utterances, and phrases in a conversation
- Created each segment for its targeted sound signal with a 200-400 milliseconds padding at the start and end of a sound signal.
- For all segments, the following objects were present and filled i.e., Start Time, End Time, Segment ID, Loudness Level (Loud, Normal, Quiet), Primary Sound Type (Speech, Babble, Music, Noise, Overlap), Language Code Speaker ID, Transcription etc.
3. Quality Check and Feedback
- All recordings were assessed for quality and only validated speech recordings with WER of 90% and TER of 90% were delivered
- Quality Checklist Followed:
» Max 15 seconds of segment length
» Transcription from specific domains, namely: Weather, different types of news, health, agriculture, education, jobs or finance
» Low background Noise
» No Audio clip off – No distortion
» Correct audio segmentation for transcription
4. Data Transcription
All spoken words, including hesitations, filler words, false starts, and other verbal tics, were captured accurately in the transcription. We also followed details transcription guidelines around capital and lowercase letters, spelling, capitalization, abbreviations, contractions, numbers,
punctuation, Acronyms, Disfluent Speech, non-speech noises etc. Moreover the Work Flow followed for Collection and Transcription is as below:
Outcome
The high-quality audio data from expert linguists will enable Indian Institute of Technology – Madras, to accurately train and build multilingual Speech Recognition models in 8 Indian languages with different dialects in the stipulated time. The Speech recognition models can be used to:
- Overcome language barrier for digital inclusion by connecting the citizens to the initiatives in their own mother tongue.
- Promotes Digital Governance
- Catalyst to form an ecosystem for services and products in Indian languages
- More localized digital content in the domains of public interest, particularly, governance & policy
We were impressed with Shaip’s expertise in conversational AI space. Their overall project execution competency from sourcing, segmenting, transcribing and delivering the required training data from expert linguists in 8 languages within stringent timelines and guidelines; while still maintaining the acceptable standard of quality.”
Featured Clients
Empowering teams to build world-leading AI products.