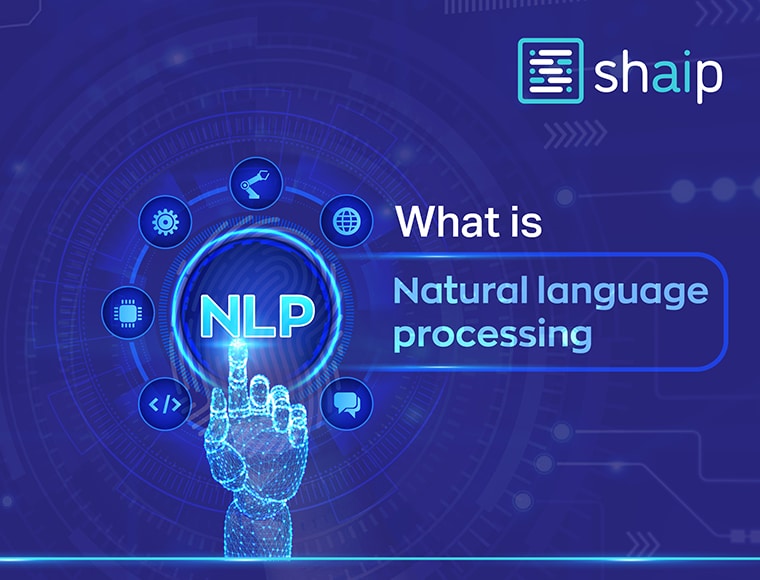
What is Natural Language Processing (NLP)?
Natural Language Processing (NLP) is a subset of Artificial Intelligence (AI) – specifically Machine Learning (ML) that allows computers and machines to understand, interpret, manipulate, and communicate human language.
One of the primary reasons systems and computers have been able to precisely mimic human communication is because of the abundant availability of data in the form of audio, texts, conversational data on social media channels, videos, emails, and more. The development of meticulous syntaxes have enabled models to accurately understand nuances in human communication including sarcasm, homonyms, humor, and more.
Some of the most basic applications of NLP include:
- Real-time language translation
- Spam filters in email services
- Voice assistants and chatbots
- Text summarization
- Autocorrect features
- Sentiment analysis and more
How Natural Language Processing (NLP) Works?
Natural Language Processing (NLP) systems use machine learning algorithms to analyze large amounts of unstructured data and extract relevant information. The algorithms are trained to recognize patterns and make inferences based on those patterns. Here's how it works:
- The user must input a sentence into the Natural Language Processing (NLP) system.
- The NLP system then breaks down the sentence into smaller parts of words, called tokens, and converts audio to text.
- Then, the machine processes the text data and creates an audio file based on the processed data.
- The machine responds with an audio file based on processed text data.
Approaches to Natural Language Processing.
Some of the approaches to NLP are:
Supervised NLP: Trains models on labeled data to make accurate predictions, like classifying emails.
Unsupervised NLP: Works with unlabeled data to find patterns, useful for tasks like topic modeling.
Natural Language Understanding (NLU): Helps machines interpret and understand the meaning of human language.
Natural Language Generation (NLG): Creates human-like text, such as writing summaries or chatbot responses. Refer more
NLP Market Size & Growth
The Natural Language Processing (NLP) market is showing phenomenal promise and is anticipated to be valued at around $156.80bn by the year 2030. This growth is at an annual CAGR of 27.55%.
Besides, over 85% of the large organizations are working on adopting NLP by the year 2025. The staggering growth of NLP is fuelled by diverse reasons such as:
- Increased incorporation of AI in products and services
- The race to provide the best customer experience
- Explosion of digital data
- The availability of low-cost cloud-based solutions
- The adoption of the technologies across diverse industries including healthcare, manufacturing, automotive and more
Such massive adoption and deployment of NLP also comes at a cost, where a report from McKinsey revealed that automation from NLP would make 8% of jobs obsolete. However, the report also claims that this would be responsible for creating 9% of the new job roles.
When it comes to the accuracy of results, cutting-edge NLP models have reported 97% accuracy on the GLUE benchmark.
Benefits of Natural Language Processing (NLP)
Increased documentation efficiency & accuracy
An NLP-generated document accurately summarizes any original text that humans can’t automatically generate. Also, it can carry out repetitive tasks such as analyzing large chunks of data to improve human efficiency.
Capability to automatically create a summary of large & complex textual content
Natural processing language can be used for simple text mining tasks such as extracting facts from documents, analyzing sentiment, or identifying named entities. Natural processing can also be used for more complex tasks, such as understanding human behaviors and emotions.
Enables personal assistants like Alexa to interpret spoken words
NLP is useful for personal assistants such as Alexa, enabling the virtual assistant to understand spoken word commands. It also helps to quickly find relevant information from databases containing millions of documents in seconds.
Enables the usage of chatbots for customer assistance
NLP can be used in chatbots and computer programs that use artificial intelligence to communicate with people through text or voice. The chatbot uses NLP to understand what the person is typing and respond appropriately. They also enable an organization to provide 24/7 customer support across multiple channels.
Performing sentiment analysis is simpler
Sentiment Analysis is a process that involves analyzing a set of documents (such as reviews or tweets) concerning their attitude or emotional state (e.g., joy, anger). Sentiment analysis can be used for categorizing and classifying social media posts or other text into several categories: positive, negative, or neutral.
Advanced analytics insights that were previously out of reach
The recent proliferation of sensors and Internet-connected devices has led to an explosion in the volume and variety of data generated. As a result, many organizations leverage NLP to make sense of their data to drive better business decisions.
Challenges with Natural Language Processing (NLP)
Misspellings
Natural languages are full of misspellings, typos, and inconsistencies in style. For example, the word “process” can be spelled as either “process” or “processing.” The problem is compounded when you add accents or other characters that are not in your dictionary.
Language Differences
An English speaker might say, “I’m going to work tomorrow morning,” while an Italian speaker would say, “Domani Mattina vado al lavoro.” Even though these two sentences mean the same thing, NLP won’t understand the latter unless you translate it into English first.
Innate Biases
Natural processing languages are based on human logic and data sets. In some situations, NLP systems may carry out the biases of their programmers or the data sets they use. It can also sometimes interpret the context differently due to innate biases, leading to inaccurate results.
Words with Multiple Meanings
NLP is based on the assumption that language is precise and unambiguous. In reality, language is neither precise nor unambiguous. Many words have multiple meanings and can be used in different ways. For example, when we say “bark,” it can either be dog bark or tree bark.
Uncertainty and False Positives
False positives occur when the NLP detects a term that should be understandable but can’t be replied to properly. The goal is to create an NLP system that can identify its limitations and clear up confusion by using questions or hints.
Training Data
One of the biggest challenges with natural processing language is inaccurate training data. The more training data you have, the better your results will be. If you give the system incorrect or biased data, it will either learn the wrong things or learn inefficiently.
NLP Tasks
“This is going great.”
A simple four-worded sentence like this can have a range of meaning based on context, sarcasm, metaphors, humor, or any underlying emotion used to convey this.
While understanding this sentence in the way it was meant to be comes naturally to us humans, machines cannot distinguish between different emotions and sentiments. This is exactly where several NLP tasks come in to simplify complications in human communications and make data more digestible, processable, and comprehensible for machines.
Some core tasks include:
Speech Recognition
This involves converting voice or audio data into texts. This process is crucial for any application of NLP that features voice command options. Speech recognition addresses the diversity in pronunciation, dialects, haste, slurring, loudness, tone and other factors to decipher intended message.
Speech Tagging
Similar to how we were taught grammar basics in school, this teaches machines to identify parts of speech in sentences such as nouns, verbs, adjectives and more. This also teaches systems to understand when a word is used as a verb and the same word is used as a noun.
Word Sense Disambiguation
This is a crucial process that is responsible for the comprehension of a sentence’s true meaning. Borrowing our previous example, the use of semantic analysis in this task enables a machine to understand if an individual uttered, “This is going great,” as a sarcastic comment when enduring a crisis.
Named Entity Recognition
When there are multiple instances of nouns such as names, location, country, and more, a process called Named Entity Recognition is deployed. This identifies and classifies entities in a message or command and adds value to machine comprehension.
Co-reference Resolution
Human beings are often very creative while communicating and that’s why there are several metaphors, similes, phrasal verbs, and idioms. All ambiguities arising from these are clarified by Co-reference Resolution task, which enables machines to learn that it literally doesn’t rain cats and dogs but refers to the intensity of the rainfall.
Natural Language Generation
This task involves the generation of human-like text from data. This could be text customized to slang, lingos, region, and more.
Why Is Natural Language Processing (NLP) Important?
Computers are very basic. They do not understand human languages. To enable machines to think and communicate as humans would do, NLP is the key.
It is through this technology that we can enable systems to critically analyze data and comprehend differences in languages, slangs, dialects, grammatical differences, nuances, and more.
While this is rudimentary, the refining of models with abundant training data will optimize results, further enabling businesses to deploy them for diverse purposes including:
- Uncovering critical insights from in-house data
- Deploying automation to simplify workflows, communications, and processes
- Personalization and hyper-personalization of experiences
- Implementing accessibility features to include differently abled people into computing ecosystems
- Fuelling innovation in niche domains such as clinical oncology, fleet management in supply-chain, data-driven decision making in autonomous cars and more
Use Cases
Intelligent document processing
This use case involves extracting information from unstructured data, such as text and images. NLP can be used to identify the most relevant parts of those documents and present them in an organized manner.
Sentiment Analysis
Sentiment analysis is another way companies could use NLP in their operations. The software would analyze social media posts about a business or product to determine whether people think positively or negatively about it.
Fraud detection
NLP can also be used for fraud detection by analyzing unstructured data like emails, phone calls, etc., and insurance databases to identify patterns or fraudulent activities based on keywords.
Language detection
NLP is used for detecting the language of text documents or tweets. This could be useful for content moderation and content translation companies.
Conversational AI / Chatbot for customer assistance
A conversational AI (often called a chatbot) is an application that understands natural language input, either spoken or written, and performs a specified action. A conversational interface can be used for customer service, sales, or entertainment purposes.
Text summarization
An NLP system can be trained to summarize the text more readably than the original text. This is useful for articles and other lengthy texts where users may not want to spend time reading the entire article or document.
Text Translation / Machine Translation
NLP is used for automatically translating text from one language into another using deep learning methods like recurrent neural networks or convolutional neural networks.
Question-Answering
Question answering (QA) is a task in natural language processing (NLP) that receives a question as input and returns its answer. The simplest form of question answering is to find a matching entry in the knowledge base and return its contents, known as “document retrieval” or “information retrieval.”
Data Redaction / personally identifiable information (PII) Redaction
One of the more specialized use cases of NLP lies in the redaction of sensitive data. Industries like NBFC, BFSI, and healthcare house abundant volumes of sensitive data from insurance forms, clinical trials, personal health records, and more.
NLP is deployed in such domains through techniques like Named Entity Recognition to identify and cluster such sensitive pieces of entries such as name, contact details, addresses, and more of individuals. Such data points are then made de-identifiable based on requirements.
Social Media Monitoring
Social media monitoring tools can use NLP techniques to extract mentions of a brand, product, or service from social media posts. Once detected, these mentions can be analyzed for sentiment, engagement, and other metrics. This information can then inform marketing strategies or evaluate their effectiveness.
Business Analytics
Business analytics and NLP are a match made in heaven as this technology allows organizations to make sense of the humongous volumes of unstructured data that reside with them. Such data is then analyzed and visualized as information to uncover critical business insights for scope of improvement, market research, feedback analysis, strategic re-calibration, or corrective measures.
Other possible use cases can be Grammer Correction, Sentiment Analysis, Spam Detection, Text Generation, Speech Recognition, NER, Part-of-speech tagging and more….
[Also Read: Top NLP Datasets to Supercharge Your Machine Learning Models]
Industries Leveraging NLP
Healthcare
NLP offers rewarding benefits to the healthcare industry such as:
- the extraction insights from medical records and analysis of unstructured data
- Improve and personalize clinical decision support systems
- Optimize responses from chatbots for seamless patient care experiences
- Monitor, predict, and mitigate adverse drug reactions and implement pharmacovigilance strategies and more
Fintech
The implications of NLP in fintech is completely different, offering benefits like:
- Seamless document processing and onboarding
- Optimize risk management and fraud detection
- Assessment of creditworthiness of individuals for financing
- Personalization of financial products in terms of tenures and premiums and more
Media & Advertising
NLP brings in a creative twist to media and advertising professionals, assisting them in:
- Content personalization and delivery of vernacular content
- Precision analysis and targeting of user personas
- Market research on trends, topics, and conversations for topical opportunities
- Ad copy development and placement optimization and more
Retail
NLP offers benefits to both customers and businesses in the retail space through:
- Precise recommendation engines
- Voice search optimization
- Location-based service suggestions
- Targeted advertising such as loyalty programs, first-time user discounts and more
Manufacturing
Industry 4.0 is incredibly complemented by the incorporation of NLP models through:
- Automated machine health monitoring and defect detection
- Real-time process analysis
- Optimizing delivery routes and schedules including fleet management
- Better worker and workplace safety through predictive analytics and more
Envisioning The Future Of NLP
While a lot is already happening in this space, tech enthusiasts are already supercharged for the possibilities with this technology in the years to come. Of all the clutter around the conversations on the future of NLP, one that stands prominent is Explainable NLP.
Explainable NLP
As crucial business decisions and customer experience strategies increasingly begin to stem from decisions powered by NLP, there comes the responsibility to explain the reasoning behind conclusions and outcomes as well.
This is what Explainable NLP will be all about, further ensuring accountability and fostering trust around AI solutions and developing a transparent ecosystem of AI fraternity.
Apart from Explainable NLP, the future of the technology would also involve:
- Vernacular mastery
- Integration with specialized technologies such as computer vision and robotics
- Use of NLP in addressing global concerns including sustainability, education, climate change and more
Conclusion
NLP is the way forward to better deliver products and services. With such prominence and benefits also arrives the demand for airtight training methodologies. Since razor-sharp delivery of results and refining of the same becomes crucial for businesses, there is also a crunch in terms of training data required to improve algorithms and models. Regulating and mitigating bias is of high priority as well.
This is where Shaip comes in to help you tackle all concerns in requiring training data for your models. With ethical and bespoke methodologies, we offer you training datasets in formats you need. Explore our offerings to find out more about us.
Frequently Asked Questions (FAQ)
1. What is Natural Language Processing (NLP)?
NLP is a branch of AI that focuses on the interaction between computers and human language. It enables machines to understand, interpret, and generate human language.
2. How does NLP work?
NLP uses algorithms to analyze language data, breaking down sentences into words, phrases, and syntax to extract meaning and perform tasks.
3. What are the benefits of NLP?
NLP improves communication between humans and machines, enhances customer service through chatbots, and aids in data analysis by processing large amounts of text data.
4. What challenges does NLP face?
Challenges include language ambiguity, context understanding, and processing non-standard language, such as slang or dialects.
5. What are some examples of NLP applications?
Examples include virtual assistants like Siri, sentiment analysis tools, and machine translation services like Google Translate.
6. How is NLP used in healthcare?
In healthcare, NLP is used for tasks like medical record analysis, automating documentation, and extracting relevant information from patient data.