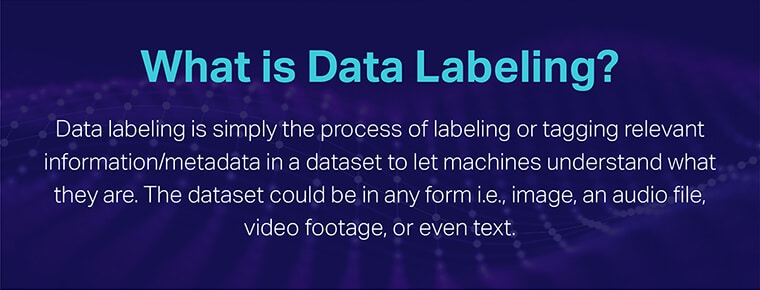
Intelligent AI models need to be trained extensively for being able to identify patterns, objects, and eventually make reliable decisions. However, the trained data cannot be fed randomly and must be labeled to help the models understand, process, and learn comprehensively from the curated input patterns.
This is where data labeling comes in, as an act of labeling information or rather metadata, as per a specific dataset, to focus on amplifying the understanding of the machines. To simply further, Data labeling selectively categorizes data, images, text, audio, videos, and patterns to improve AI implementations.
As per NASSCOM Data labeling Report, the global data labeling market is expected to grow by 700% in value by the end of 2023, as compared to that in 2018. This purported growth is most likely to factor in the financial allocation for self-managed labeling tools, internally supported resources, and even third-party solutions.
In addition to these findings, it can also be inferred that the Global Data labeling market amassed a value of $1.2 billion in 2018. However, we are expecting it to scale as the data labeling market size is presumed to reach a massive valuation of $4.4 billion by 2023.
Data labeling is the need of the hour but comes with several implementation and price-specific challenges.
Some of the more pressing ones include:
- Sluggish data preparation, courtesy of redundant cleansing tools
- Lack of requisite hardware to handle a massive workforce and excessive volume of scraped data
- Restricted access to avant-garde labeling tools and supporting technologies
- Higher cost of data labeling
- Lack of consistency when quality data tagging is concerned
- Lack of scalability, if and when the AI-model needs to cover an additional set of participants
- Lack of compliance when it comes to maintaining a steady data security posture whilst procuring data and using it
Although you can segregate data labeling conceptually, the relevant tools require you to classify the concepts according to the nature of the datasets. These include:
- Audio Classification: Comprises audio collection, segmentation, and transcription
- Image labeling: Comprising collection, classification, segmentation, and key point data labeling
- Text labeling: Involves text extraction and classification
- Video labeling: Includes elements like video collection, classification, and segmentation
- 3D labeling: Features object tracking and segmentation
Apart from the aforementioned segregation especially from a broader perspective, data labeling is divided into four types, including Descriptive, Evaluative, Informative, and Combination al However, for the sole purpose of training, data labeling is segregated as: Collection, Segmentation, Transcription, Classification, Extraction, Object Tracking, which we have already discussed for the individual datasets.
Data labeling is a detailed process and involves the following steps to categorically train AI models:
- Collecting Data Sets, via strategies i.e., in-house, open source, vendors
- Labeling Data sets as per Computer Vision, Deep learning, and NLP-specific capabilities
- Testing & evaluating produced models to determine intelligence as a part of deployment
- Satisfying acceptable model quality and eventually releasing it for comprehensive usage
The right set of data labeling tools, synonymous to a credible data labeling platform need to be selected upon keeping the following factors in mind:
- Type of intelligence you wish the model to have via defined use cases
- Quality and experience of data annotators, so that they can use the tools to precision
- Quality standards you have in mind
- Compliance-specific needs
- Commercial, open-source, and freeware tools
- Budget you can spare
In addition to the mentioned factors, you are better off keeping a note of the following considerations:
- Labeling accuracy of the tools
- Quality assurance is guaranteed by the tools
- Integration capabilities
- Security and immunization against leaks
- Cloud-based setup or not
- Quality Control management acumen
- Fail-Safes, Stop-Gaps, and Scalable prowess of the tool
- The company offering the tools
Verticals that are best served by data labeling tools and resources include:
- Medical AI: Focus areas include training diagnostic models with computer vision for improved medical imaging, minimized wait times, and minimal backlog
- Finance: Focus areas include evaluating credit risks, loan eligibility, and other important factors via text labeling
- Autonomous Vehicle or Transportation: Focus areas include NLP and Computer Vision implementation to stack models with an insane volume of training data for detecting individuals, signals, blockades, etc.
- Retail & eCommerce: Focus areas include pricing-specific decisions, improved ecommerce, monitoring buyer persona, understanding buying habits, and amplifying user experience
- Technology: Focus areas include product manufacturing, bin picking, detecting critical manufacturing errors in advance, and more
- Geospatial: Focus areas include GPS and remote sensing by select labeling techniques
- Agriculture: Focus areas include using GPS sensors, drones, and computer vision to further the concepts of precision agriculture, optimize soil and crop conditions, determine yields, and more
Still confused as to which is a better strategy to get data labeling on track, i.e., Building a self-managed setup or Buying one from a third-party service provider. Here are the pros and cons of each to help you decide better:
The ‘Build’ Apporach
Build | Buy |
---|---|
Hits:
| Hits:
|
Misses:
| Misses:
|
Benefits:
| Benefits:
|
Verdict
If you plan on building an exclusive AI system with time not being a constraint, building a labeling tool from the scratch makes sense. For everything else, buying a tool is the best approach