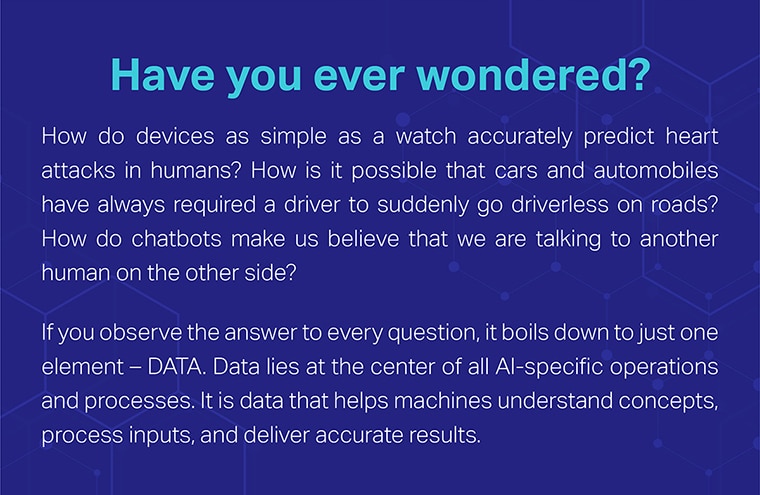
AI Data Collection: Everything You Need to Know
Intelligent AI and ML models are transforming industries, from predictive healthcare to autonomous vehicles and intelligent chatbots. But what fuels these powerful models? Data. High-quality data, and lots of it. This guide provides a comprehensive overview of data collection for AI, covering everything a beginner needs to know.
What is Data Collection for AI?
Data collection for AI involves gathering and preparing the raw data required to train machine learning models. This data can take various forms, including text, images, audio, and video. For effective AI training, the collected data must be:
- Massive: Large datasets are generally required to train robust AI models.
- Diverse: Data should represent the real-world variability the model will encounter.
- Labeled: For supervised learning, data needs to be tagged with the correct answers to guide the model’s learning.
Solution: Data Collection (Massive ammounts of data collection to train ML models.)
Acquiring AI Training Data for ML Models
Effective data collection involves careful planning and execution. Key considerations include:
- Defining Objectives: Clearly identify the goals of your AI project before starting data collection.
- Dataset Preparation: Plan for multiple datasets (training, validation, testing).
Budget Management: Establish a realistic budget for data collection and annotation. - Data Relevance: Ensure the collected data is relevant to the specific AI model and its intended use case.
- Algorithm Compatibility: Consider the algorithms you’ll be using and their data requirements.
- Learning Approach: Determine whether you’ll be using supervised, unsupervised, or reinforcement learning.
Data Collection Methods
Several methods can be used to acquire training data:
- Free Sources: Publicly available datasets (e.g., Kaggle, Google Datasets, OpenML), open forums (e.g., Reddit, Quora). Note: Carefully evaluate the quality and relevance of free datasets.
- Internal Sources: Data from within your organization (e.g., CRM, ERP systems).
- Paid Sources: Third-party data providers, data scraping tools.
Budgeting for Data Collection
Budgeting for data collection requires considering several factors:
- Project Scope: Size, complexity, type of AI technology (e.g., deep learning, NLP, computer vision).
- Data Volume: The amount of data needed depends on the project’s complexity and the model’s requirements.
- Pricing Strategy: Vendor pricing varies based on data quality, complexity, and the provider’s expertise.
- Sourcing Method: Costs will differ depending on whether data is sourced internally, from free resources, or from paid vendors.
How to Measure Data Quality?
To ensure whether the data fed into the system is high quality or not, ensure that it adheres to the following parameters:
- Intended for specific use case
- Helps make the model more intelligent
- Speeds up decision making
- Represents a real-time construct
As per the mentioned aspects, here are the traits that you want your datasets to have:
- Uniformity: Even if data chunks are sourced from multiple avenues, they need to be uniformly vetted, depending on the model. For instance, a well-seasoned annotated video dataset wouldn’t be uniform if paired with audio datasets that are only meant for NLP models like chatbots and Voice Assistants.
- Consistency: Datasets should be consistent if they want to be termed as high quality. This means every unit of data must aim at making decision-making quicker for the model, as a complementary factor to any other unit.
- Comprehensiveness: Plan out every aspect and characteristic of the model and ensure that the sourced datasets cover all the bases. For instance, NLP-relevant data must adhere to the semantic, syntactic, and even contextual requirements.
- Relevance: If you have some outcomes in mind, ensure that the data is both uniform and relevant, allowing the AI algorithms to be able to process them with ease.
- Diversified: Sounds counterintuitive to the ‘Uniformity’ quotient? Not exactly as diversified datasets are important if you want to train the model holistically. While this might scale up the budget, the model becomes way more intelligent and perceptive.
- Accuracy: Data should be free from errors and inconsistencies.
Benefits of Onboarding end-to-end AI Training Data Service Provider
Before enlisting the benefits, here are the aspects that determine the overall data quality:
- Platform used
- People involved
- Process followed
And with an experienced end-to-end service provider in play, you get access to the best platform, most seasoned people, and tested processes that actually help you train the model to perfection.
For specifics, here are some of the more curated benefits that deserve an additional look:
- Relevance: End-to-End service providers are experienced enough to only provide model and algorithm-specific datasets. Plus, they also take care of the system complexity, demographics, and market segmentation into account.
- Diversity: Certain models require truckloads of relevant datasets to be able to make decisions accurately. For instance, self-driving cars. End-to-End, experienced service providers take the need for diversity into account by sourcing even vendor-centric datasets. Put plainly, everything that might make sense to the models and algorithms is made available.
- Curated Data: The best thing about experienced service providers is that they follow a step-pronged approach to dataset creation. They tag relevant chunks with attributes for the annotators to make sense of.
- High-end Annotation: Experienced service providers deploy relevant Subject Matter Experts to annotate massive chunks of data to perfection.
- De-Identification as Per Guidelines: Data security regulations can make or break your AI training campaign. End-to-End service providers, however, take care of every compliance issue, relevant to GDPR, HIPAA, and other authorities and let you focus completely on project development.
- Zero Bias: Unlike in-house data collectors, cleaners, and annotators, credible service providers emphasize eliminating AI bias from models to return more objective results and accurate inferences.
Choosing the right Data Collection Vendor
Every AI training campaign starts with Data Collection. Or, it can be said that your AI project is often as impactful as the quality of data that is brought to the table.
Therefore, it is advisable to onboard the right Data Collection vendor for the job, who adheres to the following guidelines:
- Novelty or Uniqueness
- Timely deliveries
- Accuracy
- Completeness
- Consistency
And here are the factors you need to check as an organization for zeroing in on the right choice:
- Data Quality: Request sample datasets to assess quality.
- Compliance: Verify adherence to relevant data privacy regulations.
- Process Transparency: Understand their data collection and annotation processes.
- Bias Mitigation: Inquire about their approach to addressing bias.
- Scalability: Ensure their capabilities can scale with your project’s growth.
Ready to Get Started?
Data collection is the foundation of any successful AI project. By understanding the key considerations and best practices outlined in this guide, you can effectively acquire and prepare the data needed to build powerful and impactful AI models. Contact us today to learn more about our data collection services.
Download our infographic for a visual summary of key data collection concepts.