The growth of medical records in the healthcare industry has become both a challenge and an opportunity. Imagine a world where every detail in a patient’s medical history is not just a note in a file but a key to better healthcare. This is where AI medical records summarization steps in. It presents an opportunity to change how healthcare professionals interact with patient data.
The rise of AI in healthcare shows a transformation. Statista predicts a surge in the AI healthcare market to reach a staggering $188 billion by 2030. This leap reflects a shift towards smarter, AI-driven solutions. Medical record summarization is emerging as a tool of efficiency and precision in patient care.
What Is Summarization Of Medical Records?
Summarizing medical records is an essential process in healthcare. It involves condensing a patient’s medical history, treatments, lab reports, and notes. This task traditionally falls on doctors, nurses, and medical staff. They analyze, organize, and fill gaps in patient records. This summarization is helpful for various stakeholders in the healthcare sector.
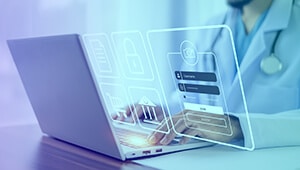
Healthcare providers
Use these summaries to merge patient data from different departments. This consolidation improves the visibility of patient information. It helps doctors make accurate diagnoses and treatment plans.
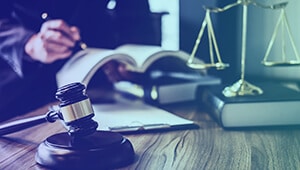
For law firms
Medical summaries provide a robust foundation for legal cases. They offer detailed accounts of a patient's medical history, treatments, and costs. These summaries strengthen the patient's legal position.
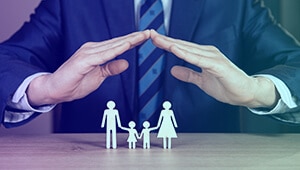
Insurance companies
Rely on medical summaries to evaluate claims. AI-generated summaries offer clear, objective data for fair patient reimbursement.
Challenges with Summarizing Medical Records
Summarizing medical records is a critical but challenging task. It requires precision and thoroughness to accurately capture all key elements of a patient’s data. Here are some of the main challenges faced in this process:
Maintaining Accuracy and Completeness
The essence of medical record summarization lies in capturing every crucial detail. This includes
- Consent for treatment
- Legal documents such as referral letters
- Discharge summaries
- Admission and clinical progress notes
- Operation notes
- Investigation reports (like X-rays or histopathology)
- Treatment orders
- Medication modification forms
- Signatures of medical professionals involved in the care
Maintaining Accuracy and Completeness
The essence of medical record summarization lies in capturing every crucial detail. This includes
Handling Voluminous Data
Medical records often contain extensive data. Sifting through this to extract relevant information is time-consuming and prone to human error.
Variability in Documentation Styles
Different healthcare providers may document the same information in various ways. This inconsistency can make summarization more complex.
Multiple Medical Formats
You’ll find summarizing medical documents complex. Medical records come in various formats, each with its own standards.
- C-CDA, or Consolidated Clinical Document Architecture, is common in the US. It uses XML to store a patient's medical history timeline.
- FHIR, or Fast Healthcare Interoperability Resources, promotes data sharing. It uses APIs for reliable data exchange across medical apps and departments.
- HL7, or Health Level 7, supports electronic health record (EHR) sharing. It utilizes messaging formats and protocols to enhance care delivery efficiency.
- SNOMED CT is a medical terminology system. It automates data processing in healthcare and ensures consistent definitions and relationships.
- ICD, or International Classification of Diseases, is a global standard. It codes diseases, injuries, and causes of death for documentation.
Interpreting Medical Jargon and Terminology
Interpreting medical jargon in record summarization requires understanding complex, specialized language. Misinterpretations can lead to errors impacting patient care and legal outcomes. This task demands professionals with medical expertise and consistent use of terminology.
Ensuring Confidentiality and Compliance
Medical records contain sensitive information. Summarizing them requires adherence to strict privacy laws and regulations, like HIPAA, in the United States.
Integrating Data from Multiple Sources
Patients often receive care from multiple providers. This results in fragmented records across various platforms and formats. It complicates the summarization process.
Example of Medical Record
Unstructured Medical Notes
John Doe, 43M, presented on 2023-07-10 with persistent headaches and dizziness for two weeks. Headaches are daily, moderate, with occasional dizziness, described as a tight band around the head. Reports mild fatigue and difficulty concentrating, especially in the afternoons. No visual changes, nausea, or vomiting. No recent trauma, illness, or changes in medication. PMH includes hypertension (2015) and seasonal allergies. Family history of migraines. Denies smoking or alcohol use. Currently on Lisinopril 20mg daily and Cetirizine 10mg PRN. Exam: BP 135/85, HR 72, neuro exam non-focal, no signs of distress. Assessment: likely tension headaches. Plan: continue current meds, use OTC NSAIDs for relief, recommend stress management techniques, follow up in 4 weeks or sooner if symptoms worsen. Advised adequate hydration and regular sleep.
Structured Medical Record
Patient: John Doe, 43-year-old male
Symptoms:
- Daily headaches (moderate, tight band sensation)
- Occasional dizziness
- Mild fatigue
- Difficulty concentrating
Medical History:
- Hypertension (since 2015)
- Seasonal allergies
- Family history of migraines
Lifestyle:
- Denies smoking or alcohol use
Current Medications:
- Lisinopril 20mg daily
- Cetirizine 10mg as needed
Physical Exam:
- Blood Pressure: 135/85 mmHg
- Heart Rate: 72 bpm
- Neurological exam: Non-focal
Diagnosis:
- Likely tension headaches
Recommendations:
- Continue current medications
- Use NSAIDs for headache relief
- Stress management techniques
- Adequate hydration and sleep
- Follow-up in 4 weeks or sooner if symptoms worsen
Best Practices for Implementing Generative AI in Medical Record Summarization
Implementing generative AI in medical record summarization offers significant potential to enhance healthcare efficiency and accuracy. However, you must follow certain best practices to maximize its benefits. Here, we explore key strategies for successful AI integration in this critical domain.
- Data Quality and Integrity: Ensure the data fed into the AI system is high quality. Accurate, complete, well-structured data can help you with effective AI training and output.
- Customized AI Models: Develop AI models tailored to specific medical contexts. Generative AI should be trained on datasets relevant to the particular medical field it will serve.
- Continuous Learning and Updating: AI models should evolve with ongoing learning. Regular updates with new medical data and terms help maintain accuracy and relevance.
- Integration with Existing Systems: Seamless integration of AI tools with existing healthcare IT systems is vital. This ensures smooth data flow and usability in clinical settings.
- Compliance with Privacy Regulations: Adhere strictly to patient privacy laws and data protection regulations. Generative AI systems must be designed to maintain confidentiality and comply with standards like HIPAA.
- User-Friendly Interface: The AI system should have an intuitive interface for ease of use by healthcare professionals. This enhances adoption and effective utilization.
- Quality Control and Oversight: Regular audits and quality checks by medical experts are essential. This ensures the AI-generated summaries are accurate and clinically valid.
- Training for Healthcare Professionals: Provide comprehensive training to healthcare staff using the AI system. Understanding its capabilities and limitations is key for effective use.
- Collaboration with Clinical Experts: Involve clinicians and medical record experts in the AI development process. Their insights ensure the AI is aligned with real-world clinical needs.
- Ethical Considerations and Bias Mitigation: Address ethical concerns and actively work to mitigate biases in AI algorithms. Ensuring fairness and representativeness in AI-generated summaries is critical.
All these best practices can help you improve patient care and operational efficiencies in the healthcare sector.
Conclusion
AI medical records summarization, powered by generative AI, revolutionizes healthcare by efficiently condensing patient histories into actionable insights.
Facing challenges like maintaining data integrity and interpreting medical jargon necessitates best practices. These include ensuring data quality, customizing AI models, and adhering to privacy regulations. This approach promises enhanced patient care and operational efficiency in the healthcare sector.