Healthcare has always been a field where innovation is appreciated and crucial for saving lives. Despite technological advancements, the healthcare industry still faces lingering challenges.
PwC says healthcare costs will rise 7% in 2024. This is due to staff burnout, insufficient workers, payment issues, and rising prices. The industry is looking at new tech to provide good care without high costs. One key area is Generative AI in healthcare.
Generative AI adds speed, improves accuracy, and opens doors for innovations that we haven’t yet imagined.
In this article, we’ll discuss the power of Generative AI in healthcare, its applications, and some ethical considerations.
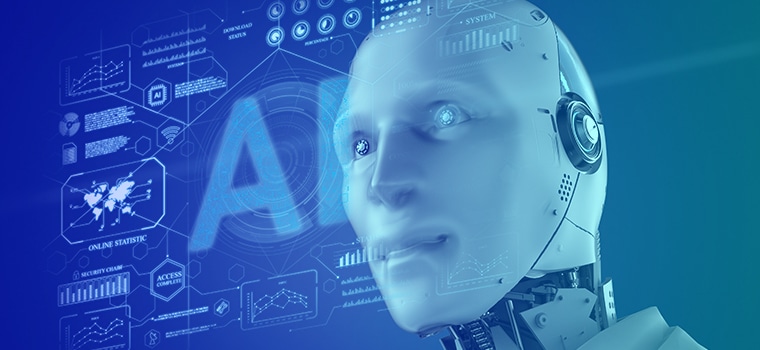
Applications of Generative AI in Healthcare
Generative AI offers solutions for training, diagnosis, drug development, and more in healthcare. Let’s look at key areas where this tech holds more importance.
Medical Training and Simulations
Generative AI creates realistic health scenarios for training. Unlike traditional methods with fixed scenarios, AI adapts in real-time. This enriches the learning experience. For example, the University of Michigan uses AI to simulate sepsis treatment, while the University of Pennsylvania studies COVID-19 spread.
Clinical Diagnosis
Generative AI helps in two main ways: enhancing medical images and diagnosing diseases. AI can turn low-quality scans into high-resolution images. It also uses patient data to detect early signs of conditions like skin cancer or Alzheimer’s. Google’s Med-Palm 2, trained on medical data, achieved an 85% accuracy rate in answering medical questions.
Drug Development
New drug development is a costly affair, often reaching billions of dollars. Generative AI can cut costs significantly. It can design new molecules and predict the properties of new drugs. Recently, Recursion Pharmaceuticals acquired Valence, an AI startup, to design drug candidates using AI.
Administrative Tasks
Doctors face a high burnout rate, partially due to administrative work. Generative AI can help automate tasks like filling electronic health records (EHRs) and scheduling appointments. Navina, an AI startup, built a tool to assist doctors in these tasks and has already secured $44 million in funding.
Synthetic Medical Data
Data for medical research is hard to come by, especially for rare diseases. Generative AI can produce synthetic data samples, bypassing privacy concerns. German researchers developed GANerAid, an AI model that generates synthetic patient data for clinical trials.
Advantages and Challenges of Generative AI in Healthcare
Advantages
- Speed: One of the most compelling benefits is how quickly tasks get done. For example, AI can sift through extensive medical records in seconds, which would take a human much longer.
- Accuracy: Generative AI excels in improving diagnosis quality. It can identify early-stage diseases with higher accuracy compared to traditional methods. For instance, AI algorithms have shown promise in early cancer detection.
- Accessibility: Generative AI can make healthcare more available. Think of rural areas where specialized care is limited. AI-powered telemedicine platforms can offer consultation and diagnosis services remotely.
Challenges
- Bias: AI models can inherit biases present in their training data. This is a concern in healthcare, where biased algorithms could lead to unequal treatment for different demographic groups. For example, a study showed that an AI used in healthcare was less accurate in diagnosing skin conditions for people with darker skin tones.
- Data Privacy: One of the biggest concerns in healthcare is the security of sensitive data. Since AI relies on large data sets, there’s always the risk of data breaches. However, a 2023 survey shows that AI can also be part of the solution. It can save organizations nearly $1.8 million in data breach costs and speed up breach identification by over 100 days.
- Implementation Costs: Setting up AI tools can be expensive. Initial costs include software development, hardware setup, and training staff to use the new systems.
- Regulation: AI’s medical applications are still a regulatory grey area. There are questions about accountability in case of misdiagnosis or data mishandling. The US and EU are making plans to bring new regulations, but this won’t happen soon.
Future Views and New Trends in GenAI in Healthcare
As generative AI continues to gain traction, we see new emerging trends that will shape the upcoming years of the healthcare industry. Here’s an overview of these trends:
Better Diagnosis and Custom Medicine
GenAI will improve disease diagnosis and enable tailored medical treatments. Future models will produce detailed medical images and identify diseases with high accuracy.
AI and Human Teamwork
GenAI will promote settings where humans and AI collaborate. Effective interaction between healthcare workers and AI is necessary for maximizing benefits.
Big Data and EHRs
Merging GenAI with big data and electronic health records is promising. These AI models can analyze diverse patient data to give helpful insights. They can use EHRs to find trends, make predictions, and fine-tune treatments.
Ongoing Learning
GenAI needs to keep learning to stay useful. It must adapt to new data, diseases, and healthcare shifts. Future models will likely be able to learn continually, making them more accurate and useful.
Role of Generative AI Data in Healthcare
Data plays an important role in powering generative AI for the healthcare industry. Here’s how:
Training Models
High-quality data is crucial for training AI algorithms. These models learn from past patient histories, medical images, and even genetic information to become smarter.
Improving Accuracy
The more diverse and extensive the data set, the better the AI model can predict and diagnose. For example, an AI trained on a broad range of X-rays can more accurately identify lung issues.
Personalized Medicine
Data allows AI to tailor treatments to individual needs. For example, AI can analyze data from multiple sources to recommend the most effective medication for a patient.
Predictive Analytics
With enough data, AI can forecast patient needs and healthcare trends. It can predict disease outbreaks or anticipate the resources a hospital will need.
Ethical and Legal Compliance
Data helps ensure that AI models comply with healthcare regulations. Proper data can help identify any biases or inaccuracies that could lead to unequal treatment.